
The field of Artificial Intelligence (AI) is constantly advancing and exploring new possibilities. Recently, at the NeurIPS conference – a gathering of AI researchers, NVIDIA presented a range of groundbreaking advancements.
This article delves into these new techniques, exploring how NVIDIA’s research is shaping the future of AI. These techniques include text generation, image processing, and even robotics.
Moreover, we will analyze the potential implications of these breakthroughs, from creative content creation to more sophisticated medical treatments.
Seimaxim offers GPU servers featuring top-tier NVIDIA Ampere A100, RTX A6000 ADA, GeForce RTX 3090, and GeForce RTX 1080Ti cards. We also provide Linux and Windows VPS options for various computing needs.
What is NeurIPS?

NeurIPS, formerly NIPS, is an annual conference on neural information processing systems. It has been held every December since its inception. The conference will showcase invited talks and oral and poster presentations of research in the field. NeurIPS 2024 will occur from December 9 to December 15 in Vancouver. Authors submit their research papers following specific formatting instructions. Submissions are limited to eight content pages.

It includes figures and tables in the NeurIPS “submission” style. Additional pages containing broader impact statements and references are allowed. The maximum file size for submissions is 50MB. It’s a major annual event, mainly focusing on the fields of:
- Machine Learning: This is the core area of NeurIPS, encompassing techniques that allow computers to learn without explicit programming.
- Computational Neuroscience: This field uses computational models and principles to explore the brain’s workings.
NVIDIA Pushes AI Boundaries with New Techniques at NeurIPS
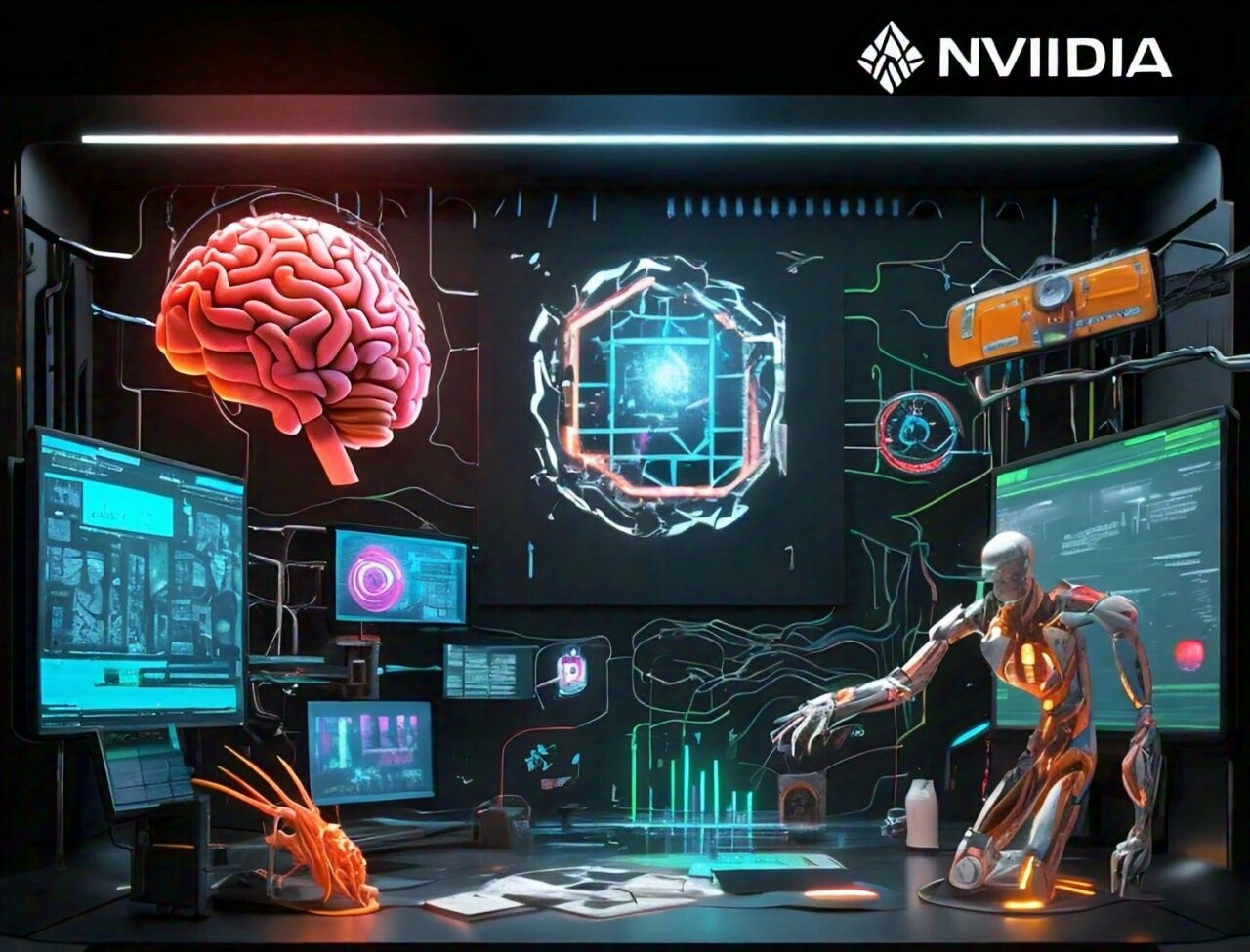
NVIDIA researchers likely presented papers or gave talks at NeurIPS showcasing their latest findings in AI. Hence, These findings might involve new algorithms or approaches that could improve the performance of AI systems in various tasks. The “boundaries” being pushed could refer to limitations in areas like text generation, image recognition, robotics, or other applications of AI.
NVIDIA’s accepted papers feature a range of groundbreaking research. Discover outstanding NeurIPS work, from GANs that create photorealistic images to semantic segmentation with transformers.
Scientific Computing: AI-Accelerated Physics, Climate, Healthcare
It could involve using AI to simulate physical phenomena or analyze medical data. NVIDIA’s team of skilled researchers will present insightful papers at the NeurIPS conference.
Hence, it will cover various topics within the natural sciences.
Their research will delve into physics simulations, climate models, and the application of AI in healthcare. Therefore, it will provide valuable insights and advancements in these crucial fields. For example, AI can predict dosage in healthcare.
Hybrid Multi-Scale Climate Simulators

The research behind ClimSim won the “Outstanding Datasets and Benchmarks Paper Award” at the NeurIPS 2023 conference, highlighting its significance in the field.
NVIDIA introduced this tool, and research in this area is likely ongoing. This method integrates conventional physics-based climate models with machine learning (ML) techniques.
Climate simulations are essential for understanding the Earth’s climate and predicting future changes. However, the resolution of the models used limits these simulations. To address this limitation, we propose creating a comprehensive dataset, ClimSim.
It can be helpful to train high-resolution physics emulators. These emulators can accurately simulate the climate at a much higher resolution than is currently possible.
Therefore, it allows for more accurate predictions of climate change and its potential environmental and societal impacts.
ClimSim

The ClimSim dataset will be created by combining data from various sources.
It includes satellite observations, ground-based measurements, and existing climate models. ClimSim can significantly advance our understanding of climate change and inform effective mitigation.
Moreover, it will give us more information about adaptation strategies by providing a more in-depth and accurate representation of the Earth’s climate.Challenges in Climate Simulation:
- Due to computational limitations, traditional climate models struggle to capture small-scale features like storms and turbulence.
ClimSim Dataset:
- This dataset is the largest ever created for training physics emulators in climate simulations.
- It consists of 5.7 billion data points simulating various atmospheric phenomena.
- It covers global climate data over multiple years with high sampling frequency.
- It is made to be compatible with existing climate models.
Benefits:
ClimSim is a tool that enables researchers to develop machine-learning emulators for high-resolution simulations of essential climate processes.
Therefore, it can produce more precise and comprehensive climate forecasts without needing expensive high-resolution simulations everywhere.
Moreover, the dataset is openly accessible, which promotes cooperation and breakthroughs in hybrid multi-scale climate simulations.
Generative AI
This field involves creating realistic and imaginative content, including images, text, and videos. Recent advancements in diffusion models have enabled the generation of images from text and long videos from text prompts.
Text-Driven Consistent Scene Generation
Diffusion models for generating realistic images from text have become increasingly popular in artificial intelligence. These models can generate high-quality photos that accurately reflect the input text.

NVIDIA, a leading technology company, has been at the forefront of research into diffusion models. Hence, they collaborate with several universities to advance technology in multiple projects.
The collaboration between NVIDIA and these universities has focused on enhancing the performance of diffusion models. Therefore, this improves their ability to generate high-quality images more closely aligned with the input text.
The results of this research will be presented at the annual Conference on Neural Information Processing Systems (NeurIPS).
The upcoming presentations at NeurIPS will showcase the latest advancements in diffusion models and their potential applications in various fields.
The research conducted by NVIDIA and its university partners has the potential to revolutionize how images are generated from text. Moreover, it could open up new opportunities for image-based applications across various industries.
SceneScape
Goal
- Create long videos of diverse scenes based on a text prompt describing the scene and the camera movements.
Challenge
- Existing methods for text-driven scene generation often struggle with geometric consistency. Therefore, It means the generated scenes might have unrealistic layouts, with objects floating in mid-air or illogical placements.
Solution
SceneScape tackles this challenge by combining two pre-trained AI models:
- The text-to-image generation model generates an initial image based on user input. It analyzes the initial image and predicts the depth information for each point, creating a 3D understanding of the scene.
- The depth prediction model uses an initial image to predict depth information for each point, creating a 3D understanding of the scene.
Innovation
The critical innovation in SceneScape lies in how it utilizes in-depth information. Here’s the process:
The text-to-image model generates the initial image based on the prompt.
The depth prediction model analyzes the initial image and creates a depth map. Therefore, it encodes the distance of each object from the camera.
As the camera moves through the scene, SceneScape continuously refines it in real time using depth information.
Hence, It ensures that the scene maintains geometric consistency throughout the video, preventing objects from appearing or disappearing unexpectedly.
Benefits
- Diverse Scene Generation: SceneScape isn’t limited to specific environments. Therefore, It can generate walkthrough videos of various scenes described in the text, from spaceships and caves to ice castles and cityscapes.
- Long and Consistent Videos: SceneScape can generate long walkthrough videos thanks to its online, real-time approach that refines the scene as the camera moves. Hence, it ensures a visually consistent experience throughout the video.
- Potential Applications: This research opens doors for various applications like:
- They are creating immersive virtual reality experiences for gaming, education, or tourism.
- It is Automating architectural design visualization by generating walkthroughs based on text descriptions.
- Moreover, it Simplifies 3D animation workflows by allowing artists to generate initial scene layouts quickly based on text prompts.
Overall, SceneScape represents a significant advancement in AI-generated content. Ensuring geometric consistency allows for creating more realistic virtual environments. Moreover, it provides believable virtual environments based on simple text descriptions.
However, as described in the paper, it’s important to note that SceneScape is designed for static scenes. Therefore, it may not ideally handle dynamic elements like moving people or vehicles.
Robotics

NVIDIA is a technology company that is at the forefront of research and development in the field of robotics. As part of its ongoing efforts, the company develops cutting-edge techniques that enable robots to learn, adapt, and control their surroundings more effectively.
In particular, NVIDIA’s research focuses on enhancing robots’ ability to perceive and understand their environment and improving their decision-making and problem-solving capabilities. It involves developing advanced machine learning algorithms and AI-driven systems that can process vast amounts of data quickly and accurately.
Moreover, NVIDIA is exploring new ways to control robots, including using natural language commands and gesture recognition. By enabling robots to understand and respond to human instructions more effectively, the company aims to make them more accessible and user-friendly.
NVIDIA’s research is paving the way for a new generation of intelligent, adaptable robots that can operate more autonomously in various environments. Whether in manufacturing, healthcare, or other industries, these advanced robots promise to transform how we live and work.
Robust Meta Reinforcement Learning

The researchers at NVIDIA have taken up an essential issue in Meta-Reinforcement Learning (MRL) – achieving high performance on tasks not encountered during the training phase.
MRL is a field of machine learning that focuses on an agent’s ability to learn from experience and adapt to new tasks. However, traditional reinforcement learning methods struggle with generalizing to new environments and tasks.
This research aims to address this challenge by proposing a novel approach that leverages a meta-learning algorithm to enable agents to learn quickly and effectively from new tasks.
The team’s findings suggest that the proposed method can significantly improve agents’ ability to generalize to new tasks. Moreover, it could have significant implications for developing more robust and adaptive AI systems.
Challenge in MRL

Conventional MRL (Meta-Reinforcement Learning) algorithms try to acquire a “meta-policy” that can promptly adapt to new tasks.
However, these techniques usually optimize for average performance across all functions. This approach can result in inadequate performance on challenging or dissimilar tasks encountered during training.
Meta-RL and its Applications

- MRL aims to train an AI agent to learn across various related tasks. It allows the agent to adapt quickly. Moreover, It will enable them to perform well on new tasks within a similar domain without extensive training for each specific task.
- Applications of MRL include:
- Robots that can learn to perform new tasks in a factory setting.
- Self-driving cars can adapt to different driving conditions and environments.
- AI agents that can play a variety of video games with different objectives.
Benefits of RoML
- Improved Robustness: By focusing on more complex tasks during training, RoML helps the agent develop a more robust meta-policy that performs well on unseen tasks, even those that are more difficult.
- Data Efficiency: RoML achieves this robustness without requiring a significant increase in training data compared to standard MRL methods.
Overall, RoML represents a significant improvement in MRL by enabling the development of more robust AI agents to adapt to new and challenging tasks effectively.
Seimaxim offers GPU servers featuring top-tier NVIDIA Ampere A100, RTX A6000 ADA, GeForce RTX 3090, and GeForce RTX 1080Ti cards. We also provide Linux and Windows VPS options for various computing needs.
AI Planning and Decision Making

NVIDIA and NeurIPS are actively researching and developing advanced AI techniques for planning and decision-making. This study area encompasses a range of methods and algorithms that enable AI agents to make informed decisions based on available data to achieve specific goals.
These techniques can help improve the accuracy and efficiency of AI systems by leveraging sophisticated machine-learning models and data-driven insights. Moreover, they allow them to operate more effectively in complex and dynamic environments.
Overall, NVIDIA’s collaboration with NeurIPS in this field holds significant promise for advancing AI capabilities and driving the development of innovative solutions that can benefit a wide range of industries and applications.
Policy Gradient for Rectangular Robust Markov Decision Processes

This research focuses on a method for training reinforcement learning agents in environments with uncertainties.Challenge in Reinforcement Learning (RL):
Standard RL algorithms train agents by assuming a fixed environment. However, real-world environments often have uncertainties or slight variations. Therefore, performance is suboptimal when the agent encounters unexpected situations during training. What are Markov Decision Processes (MDPs)?

Markov Decision Processes (MDPs) are a mathematical framework commonly used in Reinforcement Learning to model sequential decision-making problems. An MDP comprises five elements: states, actions, transition probabilities, rewards, and a discount factor.
The set of states represents the environment, and the set of actions represents the agent’s possible actions. Transition probabilities indicate the likelihood of moving from one state to another when an action is taken.
At the same time, rewards represent the immediate benefit or cost of taking a specific state. The discount factor determines the relative importance of immediate and future rewards. Through this representation, MDPs provide a way to understand how an agent can learn to make decisions in an uncertain environment.The Problem with Standard RL in Uncertain Environments:
- When trained in a fixed environment, RL agents often perform poorly when encountering unseen variations, leading to suboptimal decision-making.
Solution: Policy Gradient for Rectangular Robust MDPs (RPG) This research proposes a novel approach called RPG. Here’s the core idea:
- RPG utilizes the concept of rectangular robust MDPs to train agents that are robust to uncertainties in the environment.
- It employs a policy gradient method, a common RL technique, but specifically adapted for the rectangular robust setting.
- The method considers the worst-case scenario within the uncertainty rectangle, ensuring the agent learns a policy that performs well even in challenging situations.
Benefits of RPG:
- Improved Robustness: Agents trained with RPG are more adaptable and perform better in slightly different environments than those taught with standard RL methods.
- Efficient Training: The proposed approach achieves this robustness without requiring complex and computationally expensive methods for solving robust optimization problems, making it a more practical solution.
Overall, RPG significantly contributes to the field of RL by enabling the development of more robust agents that can effectively handle uncertainties in real-world environments.
Generalizable One-Shot Neural Head Avatar by NVIDIA

NVIDIA has made a remarkable breakthrough in 3D head avatar creation. The One-Shot Neural Head Avatar, developed by NVIDIA researchers, is a cutting-edge technology that allows the creation of 3D head avatars from a single image.
Moreover, This method eliminates the need for multiple images or 3D scanners. The One-Shot Neural Head Avatar technology is generalizable, meaning it can be easily applied to a wide range of images without extensive training on each image.
Hence, it is a breakthrough innovation to revolutionize 3D modeling and animation, making it more accessible and cost-effective.
Goal

The project involves developing a sophisticated system capable of producing a high-quality 3D head avatar from just one portrait image. The system will utilize advanced algorithms and techniques to accurately represent the subject’s head, including facial features, hair, and other distinguishing characteristics.
Moreover, the system can animate the avatar, making it capable of expressing various emotions and performing multiple actions. The animations will be lifelike and realistic, with smooth movements and accurate facial expressions.
This system has the potential to revolutionize computer graphics and animation. Therefore, creating compelling 3D content for movies, video games, and other applications is easier than ever. It could also have many practical applications, such as creating realistic avatars for virtual assistants or virtual reality environments.
Challenge
- Existing methods for head avatar creation often require the following:
- Multiple images of the same person.
- Time-consuming optimization processes tailored to a specific individual.
- Difficulty capturing intricate facial details beyond the face (hair, accessories).
Solution: Generalizable One-Shot Neural Head Avatar (GOHA)
GOHA utilizes a deep learning methodology to tackle the challenges above. The fundamental concept is as follows:
- Hybrid Representation: GOHA combines two components:
- Morphable Model: This captures the coarse 3D shape and expressions of the face.
- Feed-forward Networks: These networks predict finer details like vertex offsets for the underlying mesh. Moreover, it predicts a view-dependent texture for the entire head (including hair and accessories).
- Single Image Training: The model is trained on a large dataset of images containing various people and head poses. It allows it to generalize to unseen identities based on a single image.
- Volumetric Rendering and Super-Resolution: The final high-fidelity image of the avatar is generated by combining information from different viewpoints and applying a super-resolution technique for enhanced detail.
Conclusion
During the prestigious NeurIPS conference, NVIDIA presented advancements in next-gen neural networks, showcasing its leadership in AI research.
NVIDIA, a leading technology company, has made remarkable strides in developing next-generation neural networks. Their research breakthroughs have shown impressive progress in various areas. It includes scene generation, personalized speech synthesis, robust AI agents, and adaptable decision-making in uncertain environments.
With scene generation, NVIDIA has created incredibly realistic environments indistinguishable from real-life images. This technology can potentially revolutionize industries like film and gaming and create highly immersive virtual worlds.
NVIDIA’s personalized speech synthesis is another area of significant advancement. They have created synthetic voices that sound nearly identical to real individuals.
Hence, This technology can benefit people with speech impairments and those who work with voice-activated devices.
Regarding robust AI agents, NVIDIA has made strides in creating machines that can learn and adapt to changing conditions in real-time. This technology has the potential to be incredibly valuable in industries like healthcare, where quick and accurate decision-making is critical.
Finally, NVIDIA’s adaptable decision-making in uncertain environments can revolutionize fields like self-driving cars, where machines must make split-second decisions based on limited information.
Overall, NVIDIA’s advancements in next-generation neural networks are promising to revolutionize various industries and user experiences.
“If you’re interested, you can also read the following article related to this topic.”
Seimaxim offers GPU servers featuring top-tier NVIDIA Ampere A100, RTX A6000 ADA, GeForce RTX 3090, and GeForce RTX 1080Ti cards. We also provide Linux and Windows VPS options for various computing needs.